Non-Linear and High Dimensional Inference
3-7 October 2022 - IHP, Paris
In statistical learning and modern statistics, it is usually assumed that although data is observed in very high dimension, it actually live on — or near — a geometric structure of low dimension. The knowledge of such a low-complexity structure is of fundamental importance to improve both theoretical and computational aspects of a learning procedures.
Program
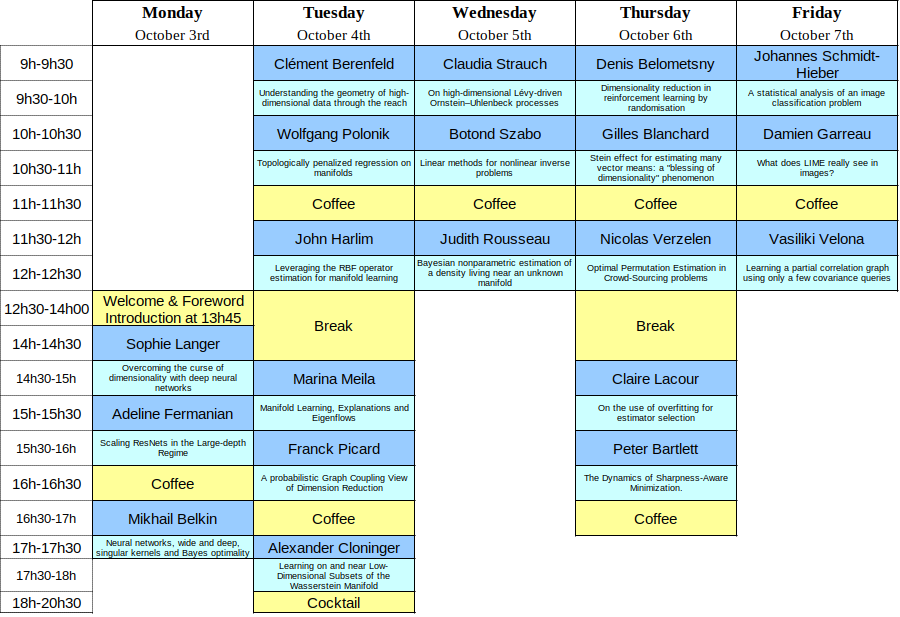
Invited Speakers
- Peter Bartlett (UC Berkeley)
- Mikhail Belkin (UC San Diego)
- Denis Belometsny (Duisburg-Essen)
- Dimensionality reduction in reinforcement learning by randomisation (video)
- Clément Bérenfeld (CEREMADE, Dauphine)
- Gilles Blanchard (LMO, Orsay)
- Alexander Cloninger (UC San Diego)
- Adeline Fermanian (Mines ParisTech)
- Damien Garreau (U Côte d'Azur)
- John Harlim (Penn State)
- Claire Lacour (Paris-Est, Marne-la-Vallée)
- Sophie Langer (TU Darmstadt)
- Marina Meila (University of Washington)
- Franck Picard (ENS Lyon)
- Wolfgang Polonik (UC Davis)
- Topologically penalized regression on manifolds (video)
- Judith Rousseau (Oxford)
- Bayesian nonparametric estimation of a density living near an unknown manifold (video)
- Johannes Schmidt-Hieber (Twente)
- Claudia Strauch (Aarhus)
- On high-dimensional Lévy-driven Ornstein–Uhlenbeck processes (slides)
- Botond Szabó (Bocconi)
- Vasiliki Velona (Hebrew University of Jerusalem)
- Nicolas Verzelen (Inrae Montpellier)
Free Afternoon & Lunch (Info handout)
- Institut Henri Poincaré’s neighborhood has plenty of diverse food places.
The two-hour lunch breaks give you enough time to eat in a restaurant, or to grab something to take away. - Wednesday afternoon is free! Feel free to stay at IHP to chat or to explore Paris.
Application
Les inscriptions pour cet événement sont actuellement ouvertes.